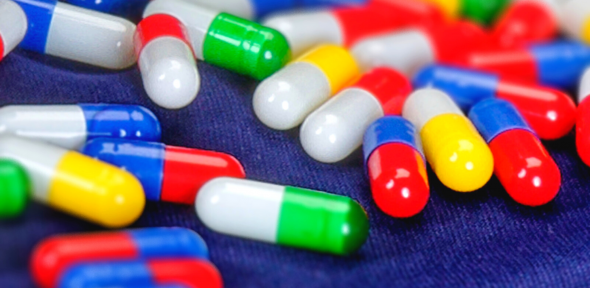
Pills, John Holman ©University of Cambridge
The Bender group, in collaboration with Uppsala University, has built a machine learning model that takes measurements from human cells and can make predictions about drug safety.
According to this study, published in Communications Biology, a machine learning model developed uses Cell Painting and gene expression data to make predictions of mitochondrial toxicity 60% better than using compound structures only. The discovery of new drugs can be a complex process, so any tools that help to accelerate the process are critical.
“Pharmaceutical companies and academics can use this model to determine whether you have mitochondrial toxicity in a compound, which is a leading cause of late-stage drug withdrawals,” says Srijit Seal, a PhD student who led the study. “This saves time and resources by identifying good, non-mitotoxic candidates before scientists start clinical tests.”
Pharmb.io Automated Lab for automated cell profiling. Pharm.Bio.
Cell Painting is when a cell is flooded with different dyes that stick to different features of the cell. This colourful cell is then profiled with a lot of detail and the results of this profiling are stored in a digital library. It is useful for general investigations and spotting patterns in large amounts of data.
The system uses data from toxic effects on mitochondria, the engine of human cells. Mitochondrial toxicity is a condition that damages the mitochondria because of which numbers decline in the cells affected. It is also possible for certain drugs to cause this toxicity as a side effect, particularly antiretroviral drugs used to treat HIV (human immunodeficiency virus). When looking for drug candidates, researchers want to limit side-effects like these as much as possible and these sophisticated tools will help identify which compounds cause mitochondria to become dysfunctional.
“In fact,” comments Seal, “We found that our models detecting mitochondrial toxicity are at par with many dedicated high content imaging assays.”
The advantage of this model is the biological data used, such as Cell Painting and gene expression. Cell Painting contains information on changes in cell structure on injecting a compound; while gene expression captures changes in 978 landmark genes. The model developed by the Bender Group spotted patterns in these data, for example, certain types of granular in the Cell Painting data indicated mitochondrial toxicity.
This model is now publicly accessible to researchers who aim to predict mitochondrial toxicity of compounds.
Winston Chiu, Ph.D. Student (Katholieke Universiteit Leuven) (left) and Srijit Seal, PhD Student (Yusuf Hamied Department of Chemistry) (right) at the SLAS conference. 2022.
Earlier this year (2022), Srijit won a Student Poster Award from the Society for Laboratory Automation and Screening (SLAS) for this research at their conference in Dublin. It recognises innovative research and, on winning the award, Seal commented:
"Our work shows that using chemical structure along with cell morphology (Cell Painting) and gene expression can greatly improve model performance and detect mitochondrial toxicity. We are using methods that can best integrate these data sources and learn much more than the training data – a process we call as expanding the applicability domain of a machine learning mode.”