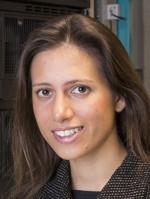
University Associate Professor
Making sense of data; how can we gain new insight and understanding from large bodies of data?
Across the natural sciences, on-going technological advances enable ever-increasing numbers of variables to be measured during single experiments. It is of crucial importance that we develop analytical techniques to extract useful information from these datasets, which are typically large and highly under-sampled, placing them outside the realm of standard statistical analysis. My research combines theory and computation to elicit structural information about relationships between variables from data.
For a given large dataset we ask about the geometry of the data - are points constrained to lie on particular manifolds, or subspaces? What metric best describes the distance between data points? Relationships between variables constrain data, resulting in correlations; statistics of the observed data can be used to infer these constraints. Visualizing these structural constraints can help to interpret their meaning, allowing us to better understand the data.
An example dataset is the set of sequences corresponding to a particular protein. The amino acid sequence contains all the information necessary to specify both the 3D structure of the protein, and the function it carries out. My work asks how this information is encoded in the sequence, and how we can exploit the large numbers of protein sequences now available to crack this code.
Protein structure and function is maintained by groups of sequence residues that mutate in a correlated fashion. Our statistical analysis of large sequence alignments exploits these correlations to make predictions of protein 3D structure and function. In this example, the dependency structure of variables is closely related to the folded protein conformation. For other questions, the physical interpretation is less straightforward, but no less important.
Projects are theoretical or computational in nature and will require interest in working across different disciplines often with experimental collaborators. Research is driven by scientific questions, which means that new analysis tools and methods are constantly being invented, developed and adopted.
Publications
- <
- 2 of 5