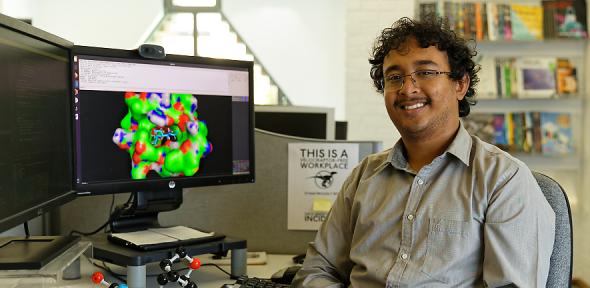
Srijit Seal in CMI, Bender group taken by Nathan Pitt ©University of Cambridge
Srijit Seal, a final-year PhD student in the Bender group, is using artificial intelligence to make drug discovery faster and safer. He has developed models that use AI to solve complex problems in medicine faster than ever.
Srijit, who is also a visiting student at the Broad Institute of MIT and Harvard, develops tools for scientists working in drug discovery. He combines drug data and AI to make predictions about how chemical compounds will interact with the body.
AI can identify patterns across vast swathes of data faster and more accurately than the human eye. These new tools will help researchers pinpoint drugs that have a higher probability of safety.
These are some of the potential applications for Srijit’s models, which he has recently published in academic journals.
Drug Cardiotoxicity
Predicting how toxic a drug would be
This AI model identifies drugs that may cause damage in the heart. Drugs can either harm the heart’s structure or alter its rhythmic functions.
Since cardiotoxicity is caused by a huge variety of factors, the model incorporates numerous datasets that record how drugs affect the heart.
“This is a new dataset released by the FDA, and this is the first work that explores how we can use AI to see patterns from a lot of different datatypes,” says Srijit.
This project has the potential to help scientists narrow down their targets when searching for new, safer drugs.
DILI PRedictor
Early Detection of Drug-Induced Liver Injury
Drug-induced liver injury can be a side effect of some medicines, and can be very dangerous. It is difficult to predict because a lot of different factors come into play around this complex organ. Srijit says: “Liver injury is one of the biggest causes of a drug on the market being withdrawn.
“Liver toxicity is very complicated and is very difficult to predict with just the chemical structure. This model takes the chemical structures and other measured properties to make predictions that can take years using traditional methods.”
BioMorph
Cell health from images
The general health and makeup of cells are critical to understanding how they respond to different drugs. BioMorph makes predictions about how a drug will generally affect the body, using two datasets which have never been combined before.
One set includes detailed cell images that label the size and shape of different features of cells such as the nucleus and mitchondria. This is combined with cell health data that records how different treatments affect cells. Together, these sets predict how a particular drug might affect cells.
Srijit says: “BioMorph groups these two datasets that have never been combined before, so is potentially more interpretive and accessible for biologists.”
Srijit says if potential users are willing to give feedback on how BioMorph validates their findings then to please contact him at seal@broadinstitute.org.
This article first appeared in Chem@Cam magazine Spring 2024 Issue 68 pg 14.