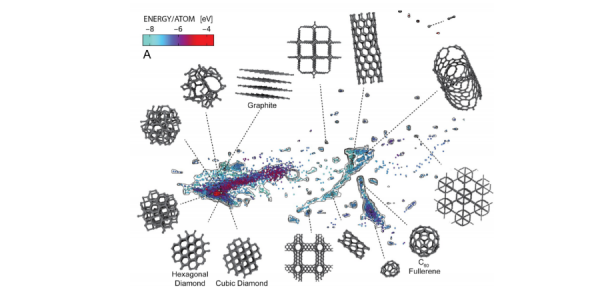
Machine learning in computational chemistry has emerged as an indispensable driver for innovation. By combining the low cost of traditional empirical potentials with the accuracy of ab-initio methods, machine learning potentials deliver long time and large length scales required for insight into complex molecular systems. Instead of physically motivated forms of interactions, the structure-energy relation is represented by highly flexible mathematical functions, such as neural networks or kernel based approaches, which are trained to reproduce high level reference calculations. Main challenges in the field are the generation of robust and accurate models, but also the construction of representative training data. Our group uses both neural network and kernel based approaches and has a strong background in automating the generation of training data to provide robust models. We use these models to shed light on complex properties such as the flow of water in nanotubes, the rippling behaviour of two-dimensional materials, or the phase behaviour of water confined in nanochannels. Common to these systems is their fine balance between inter- and intra-molecular interactions, where small errors can have drastic consequences for large-scale properties. At the same time, these systems are driven by thermal fluctuations and feature reactive processes requiring long simulations with tens of thousands of atoms. The recent advances in the field of machine learning potentials have only now opened up the door to investigate these challenging phenomena with great prospect for future work.