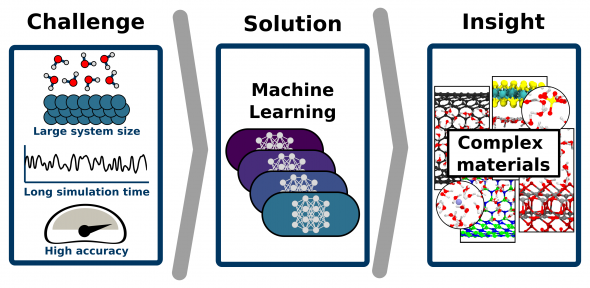
A new strategy to enable molecular simulations of complex systems has opened the door to a better understanding of complex materials.
The powerful machine-learning framework, developed by researchers from the ICE group, Imperial College London and Charles University in Prague, has been presented in the journal PNAS.
Understanding complex materials, in particular those with solid-liquid interfaces, such as water on surfaces or under confinement, is a key prerequisite in tackling some of the fundamental issues of our time, such as climate change or the lack of clean water as it enables rational material design of technologies like water nano-filtration, electrical energy storage, and heterogeneous catalyses.
Here, we show how the simple and automated machine-learning procedure can provide accurate models of these complex systems, overcoming previous limitations. To show how the models can be applied in exhaustive simulations, we successfully used the methodology on a wide set of aqueous systems with increasing degrees of complexity.
The key to the success of the procedure is that we have automated crucial steps such as the development and validation of the machine learning model. The process we have created is simple and user-friendly, where the machine learning potential is constructed with minimum human effort through a data-driven active learning protocol.
The procedure will enable more thorough and accurate investigation and understanding of complex aqueous processes such as water structuring in contact with interfaces and wetting or ice formation on surfaces.
Because it enables the fast screening of different materials at high accuracy, it should also prove useful for other materials and liquids in contact with solids, as well as general solvation phenomena.
The approach is particularly useful for situations where long timescales are involved as for the exploration of absorption phenomena, or dynamical properties, such as the friction or viscosity of liquids in contact with interfaces.
We have essentially outlined a straightforward strategy for the uncomplicated yet accurate molecular simulation of many complex systems. In addition, we made all these methods openly accessible so the new strategy can be used by researchers everywhere.
Research
Machine learning potentials for complex acqueous systems made simple, C. Schran, F. L. Thiemann, P. Rowe, E. A. Müller, O. Marsalek, A. Michaelides, PNAS (Sept 2021), https://www.pnas.org/content/118/38/e2110077118