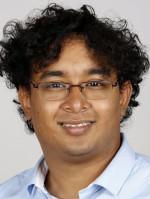
PhD, University of Cambridge
MPhil, University of Cambridge
BSc St. Stephen's College, University of Delhi
Personal Website: https://www.srijitseal.com/
For an updated list of papers, see Google Scholar.
Srijit Seal is a researcher specializing in chemoinformatics. His research uses machine learning techniques to predict drug bioactivity, safety, and toxicity, particularly modeling and interpretation of the Cell Painting assay. Seal actively engages in academic outreach, promoting the understanding of Artificial Intelligence and delivering seminars on its applications in drug discovery.
Research
For an updated list, see: Google Scholar
Predicting Cytotoxicity using Cell Painting data
We show that using Cell Painting data improved the performance of cytotoxicity models, and we can further interpret descriptors related to nuclei texture, the granularity of cells, cytoplasm and cell neighbors, and radial distributions, among others. (https://pubs.acs.org/doi/full/10.1021/acs.chemrestox.0c00303)
Predicting Mitochondrial Toxicity Using Cell Painting and Gene Expression
We perform a detailed biological interpretation of Cell Painting features in the context of mitochondrial toxicity prediction. We further show that using Cell Painting, Gene Expression features, and Morgan fingerprints significantly improves the applicability domain of structural models. (https://www.nature.com/articles/s42003-022-03763-5)
Similarity-based merger models
Designing fusion models of morphology and chemical space using a decision boundary based on their distance to the training set to improve the prediction of biological assay outcomes. (https://jcheminf.biomedcentral.com/articles/10.1186/s13321-023-00723-x)
From Pixels to Phenotypes: Interpreting Cell Painting Features in BioMorph Space
Developing a data-based standardized and comprehensive framework for understanding the complex features in the Cell Painting assay from a biological perspective. (broad.io/BioMorph, https://www.molbiolcell.org/doi/pdf/10.1091/mbc.E23-08-0298)
PKSmart: An Open-Source Computational Model to Predict in vivo Pharmacokinetics of Small Molecules
Using chemical structure and animal data, human PK prediction is used to build interpretable PK prediction models. (https://broad.io/PKSmart)
Improved Early Detection of Drug-Induced Liver Injury by Integrating Predicted in vivo and in vitro Data
We are repurposing in vitro and in vivo data to predict organ-level toxicity with interpretable machine learning. (https://broad.io/DILIPredictor)
Insights into Drug Cardiotoxicity from Biological and Chemical Data: The First Public Classifiers for FDA Drug-Induced Cardiotoxicity Rank (http://broad.io/DICTrank_Predictor, https://pubs.acs.org/doi/full/10.1021/acs.jcim.3c01834)
From pixels to phenotypes: Integrating image-based profiling with cell health data as BioMorph features improves interpretability (http://broad.io/BioMorph, https://www.molbiolcell.org/doi/full/10.1091/mbc.E23-08-0298)
Reviews
Using chemical and biological data to predict drug toxicity
In this review, we discuss the various sources of information that can be used to understand better and predict a compound's toxicity, safety, or biological activity, including using biological data such as gene expression and cell morphology. (https://www.sciencedirect.com/science/article/pii/S2472555222137147)
Current projects
Advancing the understanding of Cell Painting Representations
This work focuses on interpreting the numerical features of cell painting using convolutional neural networks to predict toxicity assays such as proliferation, oxidative stress, apoptosis, etc.
Grants
2022: Accelerate-C2D3 New Funding Programme to Help Deploy AI for Research and Innovation (as Principal Investigator leading a group of 5 others)— ~£14k to publish a review on Theoretical, Scientific, and Philosophical Perspectives on Biological Understanding in the age of Artificial Intelligence.
2024 — Winner: NIH Complement-ARIE (Promoting Non-Animal Methods) Challenge— ~ $50k for Exploring Omics for Liver Toxicity Assessment; awarded by National Institutes of Health
Awards
- Cambridge International Scholarship (2020-2023) — $188k for research during PhD starting 2020.
- BP-Clare Hall India Innovation Masters Studentship (2019) — $55k for research during MPhil starting 2019.
- Jawaharlal Nehru Memorial Fund, Honorary Allen, Meek, and Read Fund & Trinity Henry Barlow Scholarship
- Andersen-Clewell Trainee Award— $1.5k for Risk Assessment and Biological Modeling at SOT 2024.
- Royal Society of Chemistry Researcher Development Grant— ~$1.25k for SLAS Europe 2022 and SBI2 2023.
- Tony B Award and Student Poster Award— $2.5k at SLAS Europe 2022 and SLAS US 2024.
- Travel Award Summer Immersion on Innovative Approaches — $1.5k at ASCCT PCRM 2024.
- RSC Researcher Collaborations Grant — $6.5k to host Cambridge Cheminformatics Meetings, UK and US.
- Boak Fund and Progression Fund, Clare Hall (2021 and 2020 and 2022) — $5k for outstanding research.
- Pandit Hazari Lal Prize (2019) — Best student in academics, St. Stephen’s College, India.
- Japan Student Services Organization Scholarship (2018) — $1.7k
Teaching
Supervisor for Undergraduate Courses in Part IA Chemistry, University of Cambridge, UK
• Involves tutorials (220+ hours) in fundamental principles in molecular processes.
Demonstrator for Laboratory Courses in Part IA and IB Chemistry, University of Cambridge, UK
• Involves experiments (200+ hours) of organic chemistry, applications of spectroscopy and inorganic chemistry.
External Activities
• Fellow of the Cambridge Philosophical Society (2021- Life membership)
• Associate Fellow (AFHEA)Associate Fellow (AFHEA), Advance HE
• Associate Membership of the Royal Society of Chemistry (2021-)
• Former Trustee of Clare Hall, University of Cambridge (2021-22)
• Graduate Student Member, Society of Toxicology
• Graduate Student Member, British Toxicology Society
• Graduate Student Member, Society for Laboratory Automation and Screening (SLAS)
• Reviewer: Nature Machine Intelligence, SLAS Technology, SLAS Discovery, ACS Chemical Research in Toxicology, RSC Digital Discovery, Frontiers in Pharmacology, Food and Chemical Toxicology (Elsevier), ICML ML4LMS Workshop, Chemometrics and Intelligent Laboratory Systems
Industry
2024—2024 Chemoinformatics Consultant, SyzOnc, US: Building models for Gene-Compound matching and SAR analysis for internal lead compounds
2022—2023 Chemoinformatics Intern, AbsoluteAI (Ignota Labs), UK: Developing proprietary chemical structural fingerprints and models for chemical toxicity
2022—2023 Chemoinformatics Intern, PangeAI (PangeaBotanica), UK: Benchmarking and developing 3D compound fingerprints for natural products.
Publications
- 1 of 3